Chapter 12.2: Nonlinear Regression
AP Statistics Standards
I. Exploring Data:
Observing patterns and departures from patterns (continued)
D. Exploring bivariate data
-
Transformations to achieve
linearity: logarithmic and power transformations
|
Objectives |
Essential Question:
Is everything we'd like to study
and model linear? |
Chapter 12.2: 2 Variable Data Continued
Modeling Exponential Data
- Explain how data can be transformed so that
linear regression produces an exponential function.
-
First: convert all y data
points to ln y. On a
TI-83 calculator, if y-data is stored in L1 ans x-data is stored in L1, do LN
L2 sto L4
-
Second: do linear
regression for L1, L4
-
Finally: manipulate the
data as shown below
|
Time |
Microbes |
0 |
1 |
1 |
2 |
2 |
4 |
3 |
8 |
4 |
16 |
5 |
32 |
6 |
64 |
7 |
128 |
8 |
256 |
|
ln y = ax + b
e (ln y) = e[ax + b]
y = [ eax
] [ eb ]
y = [ eb
] [ eax
]
let eb
= K
y = keax
Formative Assessment: perform the transform on the above data and derive an
exponential model from it. Compare this with the linear model. Make scatter plots of both transformed and untransformed data. Are the plots concaved upward, concaved downward, or linear?
- Give examples where an exponential regression model would
be appropriate.
Growth or decay over a period of time
(response variable multiplied by a fixed
amount in each time interval) such as:
- Bacteria population vs. time
- New technologies often improve at an exponential rate. Example: the doubling of computer power every 2 years (Moore's
Law)
- New industries often go through an exponential growth spurt. Example: Growth in wind power (wind
power map)
- Radioactive decay (decay of a population of atoms)
Formative/Summative Assessment: Using the wind power links provided above and the Estimated Energy Use Sankey diagram, predict the % of total energy consumption for the United States that will be provided by wind power 10 years from today. Include your calculations and discuss your conclusions in a one-page writeup. Assume that energy consumption remains at 2012 levels.
- Explain how to determine if an exponential model is
appropriate.
Note: extrapolation can be especially risky for exponential growth models because given enough time, their output approches infinity. To use them wisely, it's necessary to consider the factors that currently are driving growth and the factors that could eventually limit further growth.
-
Explain why an
exponential model should not be selected on
the basis of optimizing r-square.
A different form of
non-linear equation may have a higher R2 value but be less appropriate.
- Perform exponential regression on a TI-83
calculator using the NON-transformed data
and note that these results and the results obtained with transformed data are
mathematically the same.
- NON-transformed data:
y = abx
- Transformed data:
y = keax
- y = k(ea)x
- Note that both k and ea are constants, so we can let: a = k and b = ea
- By substitution:
- y = abx
Homefun (formative/summative
assessment): Read section 12.2; Exercises 37 p. 788
Relevance: Exponential data is
commonplace in many business, biological, chemistry, physics, and other
areas. Knowing how to deal with it and how to model it is a significant career
skill.
|
|
Essential Question:
If
a mouse weighing 0.5 lb were scaled up by a factor of 100, how much would it
weigh? |
Modeling Power Function Data
- Explain how data can be transformed so that
linear regression produces a power function.
- First: convert all
x and y data points to ln x and ln y. On a TI-83 calculator, if
y-data is stored in L1 and x-data is stored in L1, do LN L1 sto L3 and LN L2 sto L4
- Second: do linear
regression for L3, L4
- Finally: manipulate
the data as shown below.
|
Pumpkin Dia. |
Surface Area |
1 |
1.2 |
2 |
4.7 |
3 |
9.0 |
4 |
16.9 |
5 |
25.1 |
6 |
36.5 |
7 |
49.0 |
8 |
64.9 |
|
ln y = a(lnx) + b
e (ln y) = e[a(lnx) + b]
y = [ e(lnx)a
] [ eb ]
y = [ eb
] [ xa
]
let eb
= K
y
= kxa
Formative Assessment: perform linear regression analysis and find R2.
Transform both x and y data. repeat the process. Convert the linear
regression equation to a power model.
- Give examples where a power regression model would be
appropriate.
Definition of scaling
factor:
If an object is to be scaled up to a larger size without
changing the appearance of the object, all the dimensions of the object have
to be multiplied by a common factor. This factor is called the scaling factor.
Scaling problems:
- Volume & mass
scale with the cube of the scaling factor
Note that the following volume equations all contain a cubed term.
vol of a sphere = 4/3πr3
vol of a cube with side-length of L = L3
- Area scales with the square of the scaling factor
- Explain how to determine if a power model is
appropriate.
-
Explain why a power model should not
be selected on the basis of optimizing r-square. A
different form of non-linear equation may have a higher R2 value but be
less appropriate.
- Perform power regression on a TI-83 calculator
using the NON-transformed data
and note that these results and the results obtained with transformed data are
the same.
For more information about scaling and why it's incredibly
important
-
Read: Insultingly Stupid Movie Physics
- Chapter 4, Scaling Problems: Big Bugs and Little People, pp 51 - 66
|
Relevance: Power-function data
is commonplace in many business, biological, chemistry, physics, and other
areas. Knowing how to deal with it and how to model it is a significant
career skill.
Homefun (formative/summative
assessment): Exercise 39, 43, 45 pp. 789 to 791 |
Essential Question: Can any type of non-linear data be transformed or liearized? |
Other Forms of Modeling Non-linear Data
- Describe how any power function can be linearized if the power or exponent is known.
Phenomena |
Equation |
Sample Data |
Dropped object in freefall (negligible air resistance).
g = 10 m/s2 |
y = k t2
Where:
y |
= |
distance fallen |
t |
= |
time |
k |
= |
a const. |
|
= |
1/2 g |
|
= |
5 |
|
t |
t2 |
y |
1 |
|
5.5 |
2 |
|
19.5 |
3 |
|
50 |
4 |
|
75 |
5 |
|
135 |
|
Perfect gas laws
n= 1 mole
R = 8.3 L(kPa)/(Kmol)
T = 273 K |
v = k p-1
where:
v |
= |
volume in L |
p |
= |
pressure |
k |
= |
constant |
|
= |
nRT |
|
= |
2270 (L/kPa) |
|
p |
p-1 |
v |
1 |
|
2275 |
2 |
|
1133 |
3 |
|
753 |
4 |
|
568 |
5 |
|
453 |
|
Period of a swinging pendulum
g = 10 m/s2 |
T = k L1/2
where:
T |
= |
period |
L |
= |
length |
k |
= |
constant |
|
= |
2pg1/2 |
|
= |
19.9 |
|
L |
L1/2 |
T |
1 |
|
20 |
2 |
|
28 |
3 |
|
35 |
4 |
|
40 |
5 |
|
44 |
|
Note: when performing regression analysis on the linearized data, the slope of the line equals the constant in the equation.
Formative Assessment: perform linear regression analysis and find R2 for the linearized versions of each of the above data sets. Compare the slopes to k for each data set.
Homefun (formative/summative
assessment): Exercise 33, 34 p. 786
|
Essential Question:
What is the most common form of
extrapolation? |
Interpreting Correlation and
Regression
- Decry the evils
of extrapolation but also be aware that it's commonly used.
-
projected sales-- in
order to plan ahead, companies will often attempt to predict the next
year's sales and earnings based on regression analysis of data from previous years.
-
projected population growth
-
projected impact of advertising
dollars spent -- used for determining what the
future advertising budget should be.
- radioactive dating -- there's
sound theory backing radioactive dating but obviously no one collected
data on it thousands of years ago.
Formative Assessment: Explain why real growth curves are always sigmoidal shaped (s-shaped).
- Evaluate the degree of risk associated with extrapolation.
The risks associated with extrapolation are moderated by the following:
-
Sound theoretical basis
for the regression model
-
Strong supporting data
from independent sources.For example: a limited amount of extrapolation using the
recent exponential grow in American wind-power electrical generation
is reasonable based on
ready availability of wind resources,
low cost compared to other forms of generation, and concerns about
global warming, 3 factors which make wind-power attractive.
-
Extrapolating only slightly beyond the range
of the actual data. The greater the distance
beyond the data's range, the greater the risk.
-
Simple regression model such as linear,
exponential, or power. Extrapolation with high order polynomials is
very dangerous (see example).
-
Positive results with various indicators
such as outlier-free scatter plot, high r-square, random residuals,
etc.
-
Identify
possible lurking variable. An important
variable which is not included in the study.
-
Name the most common lurking variable.
time
-
State the pitfall of using averaged
data in regression models. It makes the r-squared value higher.
Hence, the results look better than they really are.
|
Essential Question:
Can we ever be completely sure
that causation exists? |
Causation |
In other words, is the
association between the x and y variables due to the x-variable
actually causing a response in the y-variable. |
-
State 4 possible explanations for
getting a strong association based on regression/correlation analysis.
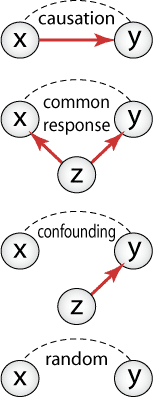
-
Causation
--Sometimes it's true: x causes y
-
Common response variables
(affect both x & y
variables), example:
rum (y) and
Methodist Ministers (x) are both affected by the common response variable,
population growth (z).
-
Confounding variables
(affect the y variable
but not the x), example:
The shaman chants an incantation (x) and five
days later the patient who seemed near death gets well. The patient's
immune system (z) was the real cause.
-
Random chance
(the association is temporary in nature and
is the result of numerous unidentifiable factors that are not
reproducible), example:
Bob finds a 1957 penny on the sidewalk as
he enters the casino. When he subsequently wins $2000 dollars at
roulette, he concludes that the penny is his good luck charm.
The dog
barked and the tree fell down.
Formative Assessment: answer the following questions:
- Did the dog cause the tree to
fall?
- Are there possible common
response variables?
- Are there possible
confounding variables?
- Could the two events coincide due
to random events?
- Could the tree-felling dog be
tested in an experiment?
- Is there a plausible theory for
why the tree could be felled by the noise of a dog barking?
|
- Explain 4 steps toward
establishing causation. Generally all 4 steps
are required especially for controversial situations.
-
Carefully controlled experiments -- the
gold standard. Can sometimes be as simple as turning the causative
variable on and off. Weakness = experiments often
are run in an artificial
environment.
-
Multiple independent observational
studies of different types
-
Account for, control, or eliminate lurking
variables -- Must be done
in both observational and experimental studies. Accounting for lurking
variables usually means including them in multiple linear regression
analysis.
-
Develop a plausible theory -- without
a plausible theory, even experimental data can be questioned.
Summative Assessment:
Test objectives 1-18 |